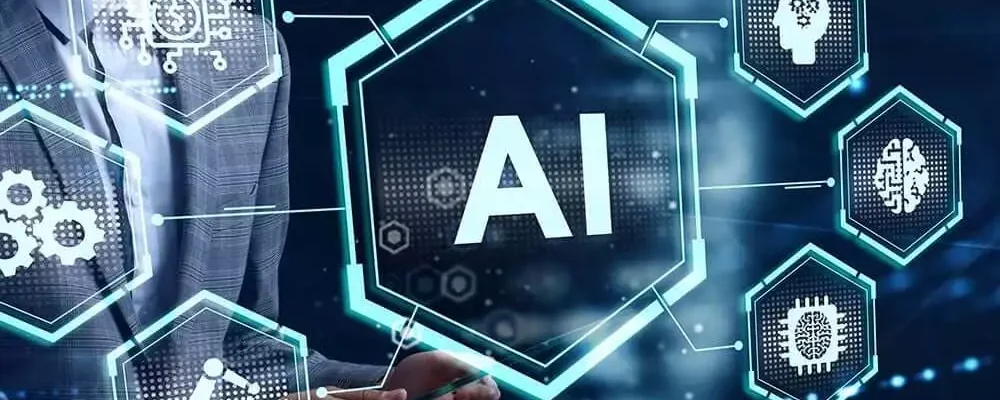
How Companies Can Ensure their AI Systems are Fair, Transparent and Accountable
January 2, 2024
Ensuring Fairness, Transparency and Accountability in AI Systems
As AI systems become more advanced and are deployed widely in society, ensuring they behave ethically and avoid unintended harms is critically important. Companies developing and using AI must address questions around the fairness, transparency and accountability of their AI systems.
Fairness refers to building AI systems that do not discriminate or disadvantage certain groups of people. For example, an AI system used for credit scoring or hiring should not make decisions based on attributes like gender, race or disability status. Achieving fairness requires identifying potential biases in training data and proactively addressing them through techniques like data augmentation, algorithmic debiasing and outcome testing.
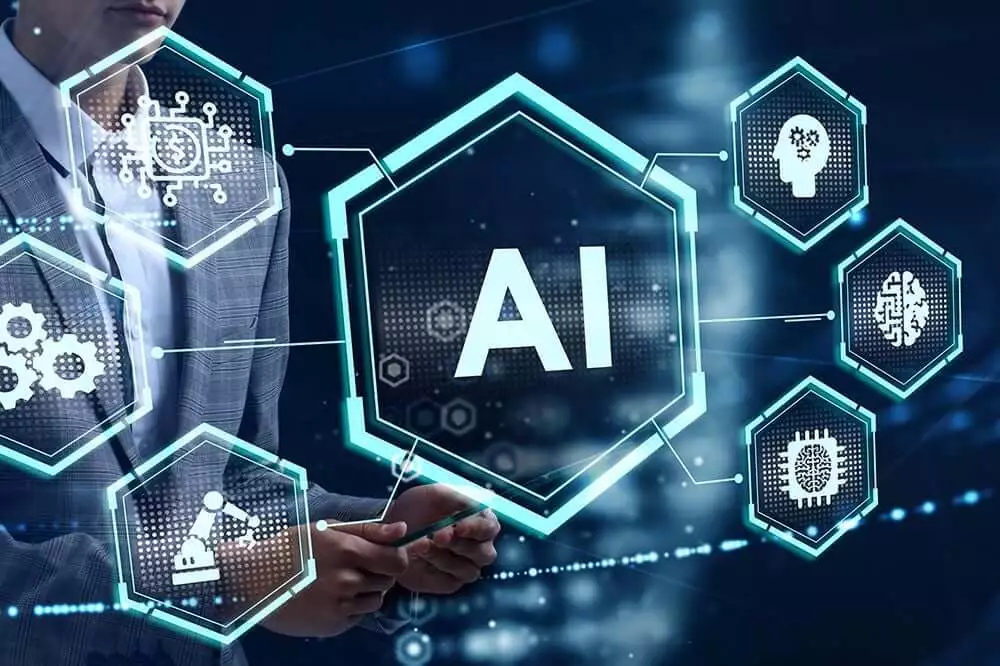
Content
Transparency deals with understanding how and why AI systems reach their conclusions. Like a “black box”, complex AI can be difficult for outsiders to comprehend. But transparency is key for establishing user trust and enabling oversight. Companies are exploring ways to open their AI systems through techniques such as model and data documentation, interactive tools and simplified result explanations.
Accountability means assigning responsibility and providing recourse when problems arise. Companies must determine governance structures for their AI systems along with processes for auditing, logging and responding to issues. They should also consider liability frameworks for high-risk applications and options for “appealing” automated decisions. Addressing accountability helps ensure appropriate oversight and consequences.
Algorithmic Fairness Assessments
Leading companies regularly evaluate their AI systems for fairness, bias and unfair or illegal discrimination according to industry standards. As reported on bestpromptaihub.com, a site dedicated to offering users ChatGPT prompts, these algorithmic fairness assessments consider metrics like statistical parity, equalized odds and calibration. Any issues uncovered are prioritized for remediation through techniques such as re-training models on more representative data or adjusting decision thresholds. Continuous monitoring and improvement is key to building trust in AI systems over time.
Model and Data Documentation
Thorough documentation of AI systems, their capabilities and limitations is another best practice. Internal documentation includes details on the system’s purpose, architecture, training methodology, data sources and potential risks. External documentation provides non-technical overviews of model performance and transparency reports on testing results. Model cards with information on functions, populations, accuracy and recommendations for appropriate use help users understand suitability. Data documentation like data sheets provide metadata to evaluate quality and fairness considerations.
Result Explanations
While complex AI may be difficult to fully comprehend, companies are exploring simplified techniques to explain automated decisions. Local explanation methods provide rationales for individual results by highlighting the most influential inputs. Global explanations give a broader view of how a model works. Interactive tools allow users to explore a model’s logic. Natural language explanations put results in plain terms people can understand. Standardizing meaningful explanations helps users trust and appropriately apply AI systems.
FAQs
What recourse do individuals have if they believe an AI decision was unfair or incorrect?
Companies should provide transparent processes for requesting re-evaluation of automated decisions and appealing results. This may involve rescoring by a human, reconsideration based on additional context, or in some regulated domains, established legal rights. Ensuring proper avenues for redress is important for accountability.
How can companies gain user trust in the ethical development and operation of AI systems?
In addition to technical measures, companies can build trust through transparency into development processes, oversight structures like advisory boards, impact assessments, certifications from independent auditors and a commitment to ongoing engagement with users and communities. Trust is built over time through consistent responsible practices.
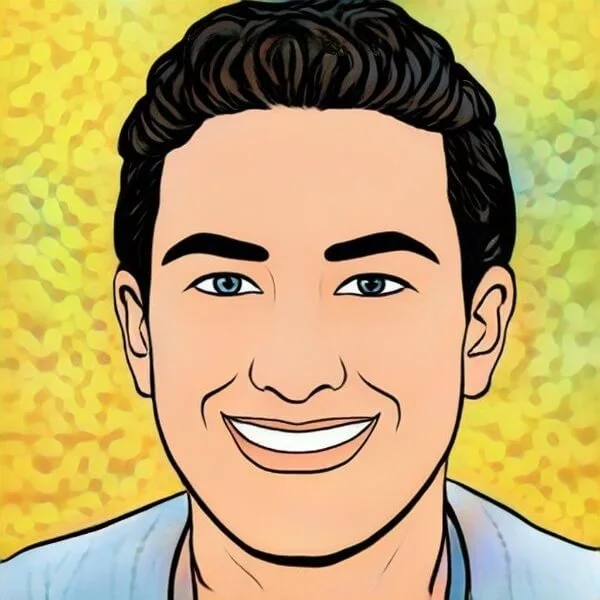
Darin is a wonderful person. He is very nice and always willing to help out! He loves his job because it lets him share interesting things with people who want to know about new developments in the world of technology.