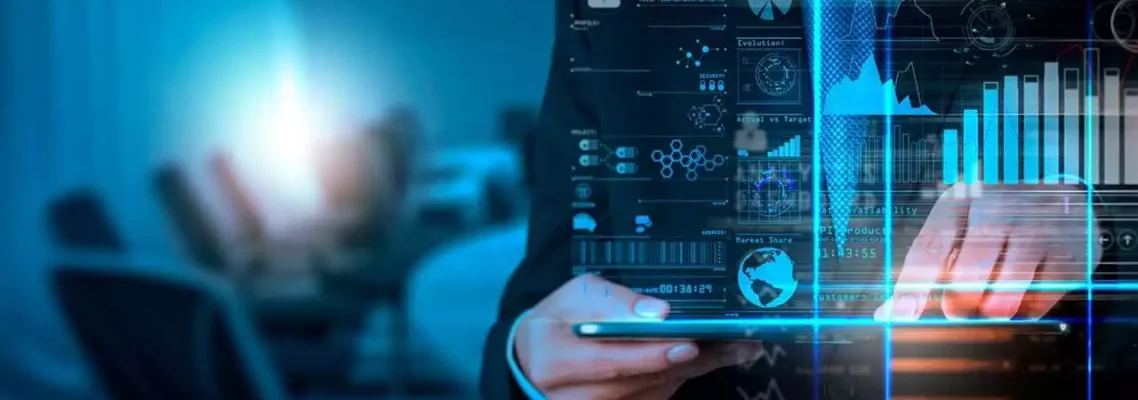
The Economics of Data Labeling: Cost-Effective Approaches for Businesses
August 8, 2023
Data labeling plays a crucial role in the development of machine learning models and artificial intelligence systems. It involves annotating raw data to provide the necessary context and information for training algorithms.
However, data labeling can be a resource-intensive and costly process for businesses, especially when dealing with large datasets. In this blog post, we’ll explore cost-effective approaches that businesses can adopt to optimize their data labeling efforts and improve their bottom line.
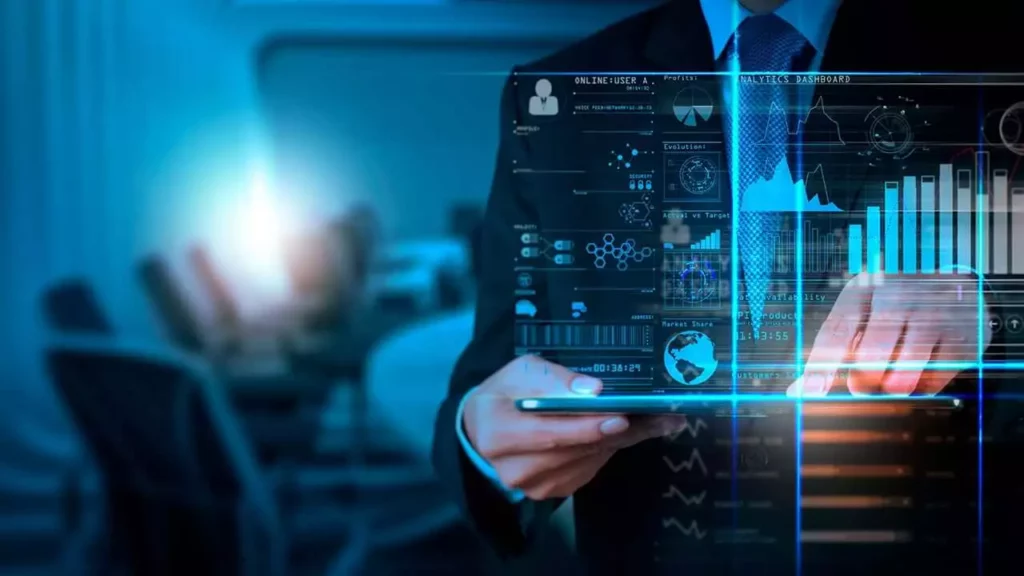
1. In-House Data Labeling Teams
Setting up an in-house data labeling team can be a smart investment for businesses with ongoing needs. By employing experts in-house, companies can save on outsourcing costs and maintain better control over the labeling process.
Additionally, having a dedicated team allows for better communication and feedback loops, leading to improved accuracy and efficiency.
2. Crowdsourcing Data Labeling
Crowdsourcing platforms can be a valuable resource for businesses seeking a cost-effective approach to data labeling. Leveraging a global workforce, companies can distribute labeling tasks to multiple contributors, reducing the time it takes to label large datasets significantly.
While crowdsourcing offers cost advantages, businesses must implement proper quality control measures to ensure accurate annotations.
3. Active Learning Strategies
Active learning is an intelligent approach that involves the selective labeling of data points. Instead of labeling the entire dataset, the model identifies the most informative samples that will enhance its learning.
By focusing on high-value data, businesses can minimize labeling costs while maximizing the model’s performance. Incorporating active learning into the process can lead to significant savings in time and resources.
4. Transfer Learning and Pre-Trained Models
Leveraging pre-trained models and transfer learning can reduce the reliance on extensive labeling for every new project. These approaches use knowledge gained from previously labeled data to accelerate the training process on new, similar tasks.
By building upon existing models, businesses can cut down on the labeling workload and speed up development without compromising accuracy.
5. Semi-Supervised Learning
Semi-supervised learning is another cost-effective method for data labeling. It combines a limited amount of labeled data with a more extensive pool of unlabeled data.
This hybrid approach reduces the need for extensive annotation while still achieving impressive results.
By leveraging the abundance of available unlabeled data, businesses can make the most of their resources.
6. Utilizing Data Labeling Tools
Investing in reliable data labeling tools can streamline the labeling process and enhance productivity. These tools often come with advanced features like automation, annotation validation, and collaborative workflows. By using such tools, businesses can speed up labeling tasks and reduce the chances of errors, ultimately saving time and money.
Conclusion
Data labeling is a fundamental aspect of AI development, but it doesn’t have to be a costly burden for businesses. By adopting these cost-effective approaches, companies can optimize their data labeling efforts and ensure efficient, accurate, and affordable training of machine learning models.
Whether through in-house teams, crowdsourcing, active learning, or leveraging pre-trained models, smart economic strategies can make data labeling more manageable and drive overall business success.
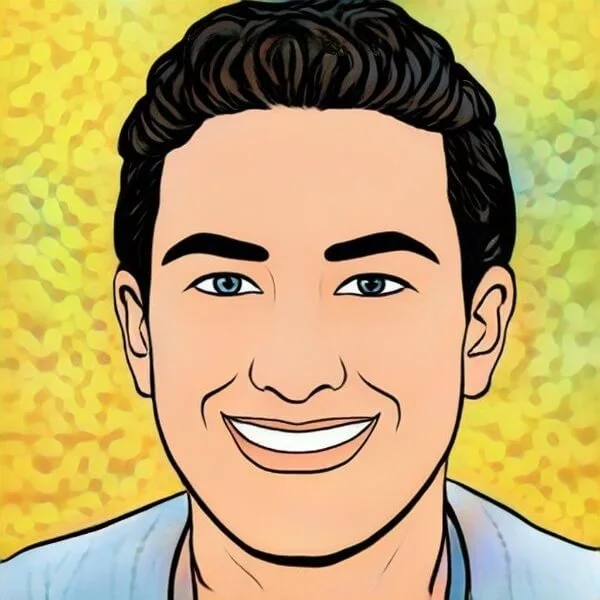
Darin is a wonderful person. He is very nice and always willing to help out! He loves his job because it lets him share interesting things with people who want to know about new developments in the world of technology.